* 본 포스트는 개인연구/학습 기록 용도로 작성되고 있습니다.
[Python] English preprocessing
By MK on July 9, 2019
영어에 대한 전처리는 대표적으로 nltk
를 사용한다.
이중에서 데이터 분석에 가장 큰 의미를 갖는 형태소인 명사
만을 추출해 실제로 데이터 분석에 활용한다.
사용 목적이나 데이터 처리 상태에 따라 다른 형태소를 포함해볼수도 있을 것이다.
명사를 추출하기 전에 추출 대상에서 제거할 불용어 사전을 미리 만들어 쓸 수도 있는데, 리스트 변수를 활용해 제거 후 추출한다.
import nltk
import pickle
from nltk.corpus import stopwords
import re
nltk.download('all')
1. 분석할 텍스트 읽어오기
with open('nytimes.txt', 'r', encoding='utf8') as f:
content = f.read()
2. 불필요한 심볼 없애기
# String function인 replace를 사용하거나 re를 사용
# cleaned_content = content.replace('!', '').replace(',','').replace('.','').replace('“','').replace('”','').replace('\n','').replace('’','')
cleaned_content = re.sub(r'[^\.\?\!\w\d\s]','',content) # 문장단위로 끊기
print(cleaned_content)
3. Case conversion
대문자를 소문자로 전환한다.
cleaned_content = cleaned_content.lower()
4. Word tokenization
각각의 워드를 토큰으로 쪼갠다.
word_tokens = nltk.word_tokenize(cleaned_content)
print(word_tokens)
['hurray', 'for', 'the', 'hotblack', 'coffee', 'cafe', 'in', 'toronto', 'for', 'declining', 'to', 'offer', 'wifi', 'to', 'its', 'customers', '.', 'there', 'are', 'other', 'such', 'cafes', 'to', 'be', 'sure', 'including', 'seven', 'of', 'the', 'eight', 'new', 'york', 'city', 'locations', 'of', 'café', 'grumpy', '.', 'but', 'its', 'hotblacks', 'reason', 'for', 'the', 'electronic', 'blackout', 'that', 'is', 'cause', 'for', 'hosannas', '.', 'as', 'its', 'president', 'jimson', 'bienenstock', 'explained', 'his', 'aim', 'is', 'to', 'get', 'customers', 'to', 'talk', 'with', 'one', 'another', 'instead', 'of', 'being', 'buried', 'in', 'their', 'portable', 'devices', '.', 'its', 'about', 'creating', 'a', 'social', 'vibe', 'he', 'told', 'a', 'new', 'york', 'times', 'reporter', '.', 'were', 'a', 'vehicle', 'for', 'human', 'interaction', 'otherwise', 'its', 'just', 'a', 'commodity', '.', 'what', 'a', 'novel', 'idea', '!', 'perhaps', 'mr.', 'bienenstock', 'instinctively', 'knows', 'what', 'medical', 'science', 'has', 'been', 'increasingly', 'demonstrating', 'for', 'decades', 'social', 'interaction', 'is', 'a', 'critically', 'important', 'contributor', 'to', 'good', 'health', 'and', 'longevity', '.', 'personally', 'i', 'dont', 'need', 'researchbased', 'evidence', 'to', 'appreciate', 'the', 'value', 'of', 'making', 'and', 'maintaining', 'social', 'connections', '.', 'i', 'experience', 'it', 'daily', 'during', 'my', 'morning', ...]
5. POS tagging
품사를 분리한다.
영어의 경우는 nltk에서 제공하는 pos_tag() 함수를 사용해서 품사 태깅을 할 수 있다.
# pos_tag()의 입력값으로는 단어의 리스트가 들어가야 한다.
tokens_pos = nltk.pos_tag(word_tokens)
print(tokens_pos)
[('hurray', 'NN'), ('for', 'IN'), ('the', 'DT'), ('hotblack', 'NN'), ('coffee', 'NN'), ('cafe', 'NN'), ('in', 'IN'), ('toronto', 'NN'), ('for', 'IN'), ('declining', 'VBG'), ('to', 'TO'), ('offer', 'VB'), ('wifi', 'NN'), ('to', 'TO'), ('its', 'PRP$'), ('customers', 'NNS'), ('.', '.'), ('there', 'EX'), ('are', 'VBP'), ('other', 'JJ'), ('such', 'JJ'), ('cafes', 'NNS'), ('to', 'TO'), ('be', 'VB'), ('sure', 'JJ'), ('including', 'VBG'), ('seven', 'CD'), ('of', 'IN'), ('the', 'DT'), ('eight', 'CD'), ('new', 'JJ'), ('york', 'NN'), ('city', 'NN'), ('locations', 'NNS'), ('of', 'IN'), ('café', 'NN'), ('grumpy', 'NN'), ('.', '.'), ('but', 'CC'), ('its', 'PRP$'), ('hotblacks', 'NNS'), ('reason', 'NN'), ('for', 'IN'), ('the', 'DT'), ('electronic', 'JJ'), ('blackout', 'NN'), ('that', 'WDT'), ('is', 'VBZ'), ('cause', 'NN'), ('for', 'IN'), ('hosannas', 'NN'), ('.', '.'), ('as', 'IN'), ('its', 'PRP$'), ('president', 'NN'), ('jimson', 'NN'), ('bienenstock', 'NN'), ('explained', 'VBD'), ('his', 'PRP$'), ('aim', 'NN'), ('is', 'VBZ'), ('to', 'TO'), ('get', 'VB'), ('customers', 'NNS'), ('to', 'TO'), ('talk', 'VB'), ('with', 'IN'), ('one', 'CD'), ('another', 'DT'), ('instead', 'RB'), ('of', 'IN'), ('being', 'VBG'), ('buried', 'VBN'), ('in', 'IN'), ('their', 'PRP$'),...]
6. 명사만 추출하기
품사 정보는 아래 링크에서 확인할 수 있다.
https://www.ling.upenn.edu/courses/Fall_2003/ling001/penn_treebank_pos.html
# 명사는 NN을 포함하고 있음을 알 수 있음
NN_words = []
for word, pos in tokens_pos:
if 'NN' in pos:
NN_words.append(word)
print(NN_words)
['hurray', 'hotblack', 'coffee', 'cafe', 'toronto', 'wifi', 'customers', 'cafes', 'york', 'city', 'locations', 'café', 'grumpy', 'hotblacks', 'reason', 'blackout', 'cause', 'hosannas', 'president', 'jimson', 'bienenstock', 'aim', 'customers', 'devices', 'vibe', 'york', 'times', 'vehicle', 'interaction', 'commodity', 'idea', 'bienenstock', 'science', 'decades', 'interaction', 'contributor', 'health', 'longevity', 'evidence', 'value', 'connections', 'experience', 'morning', 'walk', 'women', 'swim', 'locker', 'room', 'ymca', 'use', 'devices', 'locker', 'room', 'experience', 'friends', 'i', 'share', 'joys', 'sorrows', 'women', 'problems', 'board', 'advice', 'counsel', 'laugh', 'brightens', 'day', 'studies', 'life', 'harvard', 'health', 'watch', 'dozens', 'studies', 'people', 'relationships', 'family', 'friends', 'community', 'health', 'problems', 'longer', 'study', 'men', 'women', 'county', 'calif.', 'lisa', 'f.', 'berkman', 'syme', 'people', 'others', 'times', 'nineyear', 'study', 'people', 'ties', 'robbins', 'book', 'health', 'longevity', 'difference', 'survival', 'peoples', 'age', 'gender', 'health', 'practices', 'health', 'status', 'fact', 'researchers', 'ties', 'lifestyles', 'obesity', 'lack', 'exercise', 'ties', 'living', 'habits', 'robbins',...]
7. Lemmatization(원형(lemma) 찾기)
영어는 각 word의 원형을 찾는 기능을 활용할 수 있다.
원형을 찾아 같은 의미의 단어 토큰들을 하나의 값으로 인지하도록 한다.
자세한 내용은 아래 링크에서 확인할 수 있다.
https://textminingonline.com/dive-into-nltk-part-iv-stemming-and-lemmatization
# nltk에서 제공되는 WordNetLemmatizer을 이용
# ex) 명사의 경우는 보통 복수 -> 단수 형태로 변형
wlem = nltk.WordNetLemmatizer()
lemmatized_words = []
for word in NN_words:
new_word = wlem.lemmatize(word)
lemmatized_words.append(new_word)
print(lemmatized_words)
['hurray', 'hotblack', 'coffee', 'cafe', 'toronto', 'wifi', 'customer', 'cafe', 'york', 'city', 'location', 'café', 'grumpy', 'hotblacks', 'reason', 'blackout', 'cause', 'hosanna', 'president', 'jimson', 'bienenstock', 'aim', 'customer', 'device', 'vibe', 'york', 'time', 'vehicle', 'interaction', 'commodity', 'idea', 'bienenstock', 'science', 'decade', 'interaction', 'contributor', 'health', 'longevity', 'evidence', 'value', 'connection', 'experience', 'morning', 'walk', 'woman', 'swim', 'locker', 'room', 'ymca', 'use', 'device', 'locker', 'room', 'experience', 'friend', 'i', 'share', 'joy', 'sorrow', 'woman', 'problem', 'board', 'advice', 'counsel', 'laugh', 'brightens', 'day', 'study', 'life', 'harvard', 'health', 'watch', 'dozen', 'study', 'people', 'relationship', 'family', 'friend', 'community', 'health', 'problem', 'longer', 'study', 'men', 'woman', 'county', 'calif.', 'lisa', 'f.', 'berkman', 'syme', 'people', 'others', 'time', 'nineyear', 'study', 'people', 'tie', 'robbins', 'book', 'health', 'longevity', 'difference', 'survival', 'people', 'age', 'gender', 'health', 'practice', 'health', 'status', 'fact', 'researcher', 'tie', 'lifestyle', 'obesity', 'lack', 'exercise', 'tie', 'living', 'habit', 'robbins', 'people', 'lifestyle', 'tie', 'study', ...]
8. Stopwords removal
nltk에서 제공하는 불용어 사전을 통해 사용하지 않을 단어를 제거할 수 있다. 불용어 기본 사전을 통해 1차 제거용으로 활용한다.
stopwords_list = stopwords.words('english') #nltk에서 제공하는 불용어사전 이용
#print('stopwords: ', stopwords_list)
unique_NN_words = set(lemmatized_words)
final_NN_words = lemmatized_words
# 불용어 제거
for word in unique_NN_words:
if word in stopwords_list:
while word in final_NN_words: final_NN_words.remove(word)
아래와 같이 직접 불용어를 정의할 수도 있다.
# 실제 작업시에는 txt 파일로 작업하는 걸 추천
customized_stopwords = ['be', 'today', 'yesterday', "it’s", "don’t"] # 직접 만든 불용어 사전
unique_NN_words1 = set(final_NN_words)
for word in unique_NN_words1:
if word in customized_stopwords:
while word in final_NN_words: final_NN_words.remove(word)
print(final_NN_words)
['hurray', 'hotblack', 'coffee', 'cafe', 'toronto', 'wifi', 'customer', 'cafe', 'york', 'city', 'location', 'café', 'grumpy', 'hotblacks', 'reason', 'blackout', 'cause', 'hosanna', 'president', 'jimson', 'bienenstock', 'aim', 'customer', 'device', 'vibe', 'york', 'time', 'vehicle', 'interaction', 'commodity', 'idea', 'bienenstock', 'science', 'decade', 'interaction', 'contributor', 'health', 'longevity', 'evidence', 'value', 'connection', 'experience', 'morning', 'walk', 'woman', 'swim', 'locker', 'room', 'ymca', 'use', 'device', 'locker', 'room', 'experience', 'friend', 'share', 'joy', 'sorrow', 'woman', 'problem', 'board', 'advice', 'counsel', 'laugh', 'brightens', 'day', 'study', 'life', 'harvard', 'health', 'watch', 'dozen', 'study', 'people', 'relationship', 'family', 'friend', 'community', 'health', 'problem', 'longer', 'study', 'men', 'woman', ...]
9. 빈도분석
from collections import Counter
c = Counter(final_NN_words) # input type should be a list of words (or tokens)
print(c)
k = 20
print(c.most_common(k)) # 빈도수 기준 상위 k개 단어 출력
10. 워드클라우드
import wordcloud
from wordcloud import WordCloud
import matplotlib.pyplot as plt
from os import path
FONT_PATH = 'C:/Windows/Fonts/malgun.ttf' # For Korean characters
noun_text = ''
for word in final_NN_words:
noun_text = noun_text +' '+word
wordcloud = WordCloud(max_font_size=60, relative_scaling=.5, font_path=FONT_PATH).generate(noun_text) # generate() 는 하나의 string value를 입력 받음
plt.figure()
plt.imshow(wordcloud, interpolation='bilinear')
plt.axis("off")
plt.show()
워드클라우드 옵션을 변경해 볼 수도 있다. max_font_size를 변경하고 background_color를 white로 바꿔보자. max_words를 통해 최대로 표현할 단어수를 제한할 수도 있다.
wordcloud = WordCloud(max_font_size=50, max_words=30, background_color='white', relative_scaling=.5, font_path=FONT_PATH).generate(noun_text)
plt.figure()
plt.imshow(wordcloud, interpolation='bilinear')
plt.axis("off")
plt.show()
이미지를 파일로 저장하기
wordcloud.to_file("img/first_review.png")
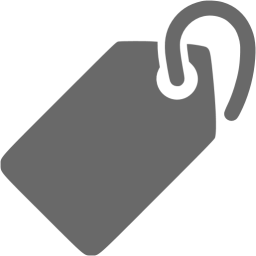